AI application example Does AI save time in political analyses?
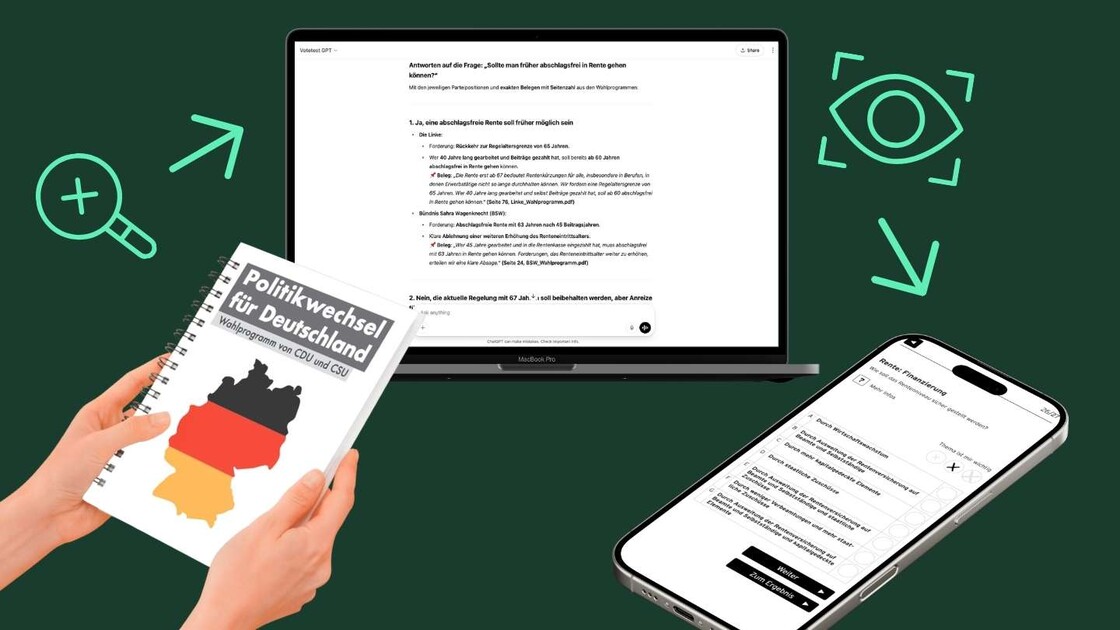
Large language models such as ChatGPT are getting better and better at processing political information. Nevertheless, when summarising statements, they are still imprecise to inadmissibly abbreviated or the information sought is hallucinated. Human control therefore remains essential at present (and probably for a very long time to come). But how much time can the use of AI save? Is it even worth using it?
We were able to test this at the beginning of 2025 on the occasion of the early Bundestag elections: Since 2001, we have been offering Votetest, a tool for playfully comparing election programmes.
Programme analysis with the election test
We search the election programmes of the approx. 5-7 parties already represented in parliament for comparable proposals. If we only find these for some of the parties, we search the Internet for corresponding statements from the other parties. These can be party conference resolutions, parliamentary initiatives or statements made by their leading figures in interviews. The next step is to summarise similar positions in terms of content. The aim is to summarise the positions of the parties not in the wording, but as short answers to a question. This does not have to be one answer per question, but also not necessarily just a yes/no answer (as in the Wahl-O-Mat). Differences in details can be ignored, but politically significant differences must be preserved and reflected in the answers.
This gave us a combination of different analytical tasks that we have completed three times in recent years in a comparable form - ideal conditions for using ChatGPT instead of our editorial team for the task this time and then comparing them. For this purpose, we used a paid ChatGPT account for two months for 40 USD. This allows you to create your own GPT, ‘Votetest GPT’ in our case, and feed it with the seven party programmes.
Pre-training
As we were not doing the previous voting test analyses for the first time, we also pre-trained the GPT voting test a little. This was also necessary for another reason: Due to the early election date, some parties only adopted their programmes quite shortly before the election. We didn't want to waste the little time available for decision-making by searching for the best prompts. We therefore initially configured our GPT vote test for the 2024 European elections, the last conventional programme comparison. This allowed us to compare the results with those of our editorial team in the previous year when optimising the prompts.
The qualitative result
Firstly, we had to sensitise Chat GPT to clearly distinguish between values or vague descriptions of objectives and concrete demands and measurable goals. Statements such as ‘We want justice to finally prevail in Germany again’ are unlikely to reveal any differences - after all, who doesn't want that? Defining this clearly, having the GPT vote test search for concrete demands and storing this in a basic prompt worked quite well.
However, the storage in the basic prompt did not always work: Although the requirement to cite sources for programme statements in the form of page numbers in the PDF was clearly formulated here, ChatGPT often forgets to cite these sources. The instruction was valid - ChatGPT confirms this with a correct response to the input ‘Repeat what I understand by source requirement’. The AI then promises to do better the next time, only to forget to cite sources again in 1/3 of all cases.
The source citation is important in order to be able to quickly check whether the statement found by the votetest GPT is also true in context. This is one of the most significant simplifications, even if the page number is also hallucinated in around one in ten cases.
This goes hand in hand with ChatGPT's current tendency to hallucinate if you insist stubbornly enough on an answer. It would have to be checked whether the choice of a more source-orientated AI such as Perplexity would offer an improvement.
It was reassuring that the case where an existing answer was not found in the programmes could largely be ruled out in the pre-training. Exceptions were passages with strong semantic deviations, cases in which, however, humans would also have problems finding the statement.
Two prompts formed the core of the work: the results of the prompt ‘Search the programmes of the parties for matching demands on the topic ####’ were quite useful. This involves moving from the main topic to sub-topics. The clearer the differences can be worked out on the basis of the party positions, the better. The decision on which aspects to focus on was not even left to the AI.
Once the sub-topic had been determined, the GPT vote test could be asked for suggestions on how to organise the positions into the desired question-answer scheme. The results were generally only useful as inspiration at best.
Once you had decided on a question, the prompt ‘Please formulate answers to the question “######?” and assign the party positions to the answers, naming the relevant demands’ proved to be a great help - as long as the sources were named. However, parties that could no longer be found in the question-answer scheme were omitted without further ado. In around half of the cases, the AI was nevertheless able to supplement answers by searching the Internet. It would have taken many times longer to research these with the help of search engines, as the AI can consider the relevance of the source in terms of the question more efficiently than a simple search engine.
The quantitative result
This brings us back to the initial question. Does this save time on the bottom line?
A distinction must be made between two values. The net time expenditure with the trained model and the gross time expenditure including preparation using the European election data. The latter is relevant if you consider it unlikely that the current configuration would simply be used again in the same way next time.
There is also a gross and a net value for human input. The gross value includes an estimated effort in personnel planning, which is not reflected in the pure hourly bookings of the editors:
The results at a glance:
Gross | Net | |||
Bundestagswahl 2021 | 65:45 h | 62:30 h | ||
Présidentielles 2022 (additional workload: SciencesPo working students) | 91:30 h | 79:00 h | ||
European Parliament election 2024 (additional workload: Team change) | 191:15 h | 156:45 h | ||
Average | 116:10 h | 99:25 h | ||
Bundestag election 2025 (additional effort: GPT training) | 46:45 h | 31:15 h | ||
Time savings | 60% | 68% |
Conclusion:
In the present case, the use of a pre-trained GPT significantly reduced the workload in both net and gross terms.
The differences are so great that even if it were unrealised that prompting and supervision of AI results requires a higher level of qualification than the previous editorial processing, the use of AI would remain more economical both in the net and in the gross view. Above all, however, the use of AI enables faster processing - and therefore a faster reaction time in the political debate.
One disadvantage remains, however. During editorial development, it is necessary to read the programmes at least cursorily from beginning to end. This gives you a different feeling and understanding of the programme, the coherence of the argumentation and the guiding principles that run through the programme. In contrast to the atomised view of set pieces through the lens of AI. At least for those working on the programme, a piece of knowledge is lost as a result.